Tuan-Anh Bui
Machine Learning Researcher in Generative AI and Trustworthy Machine Learning
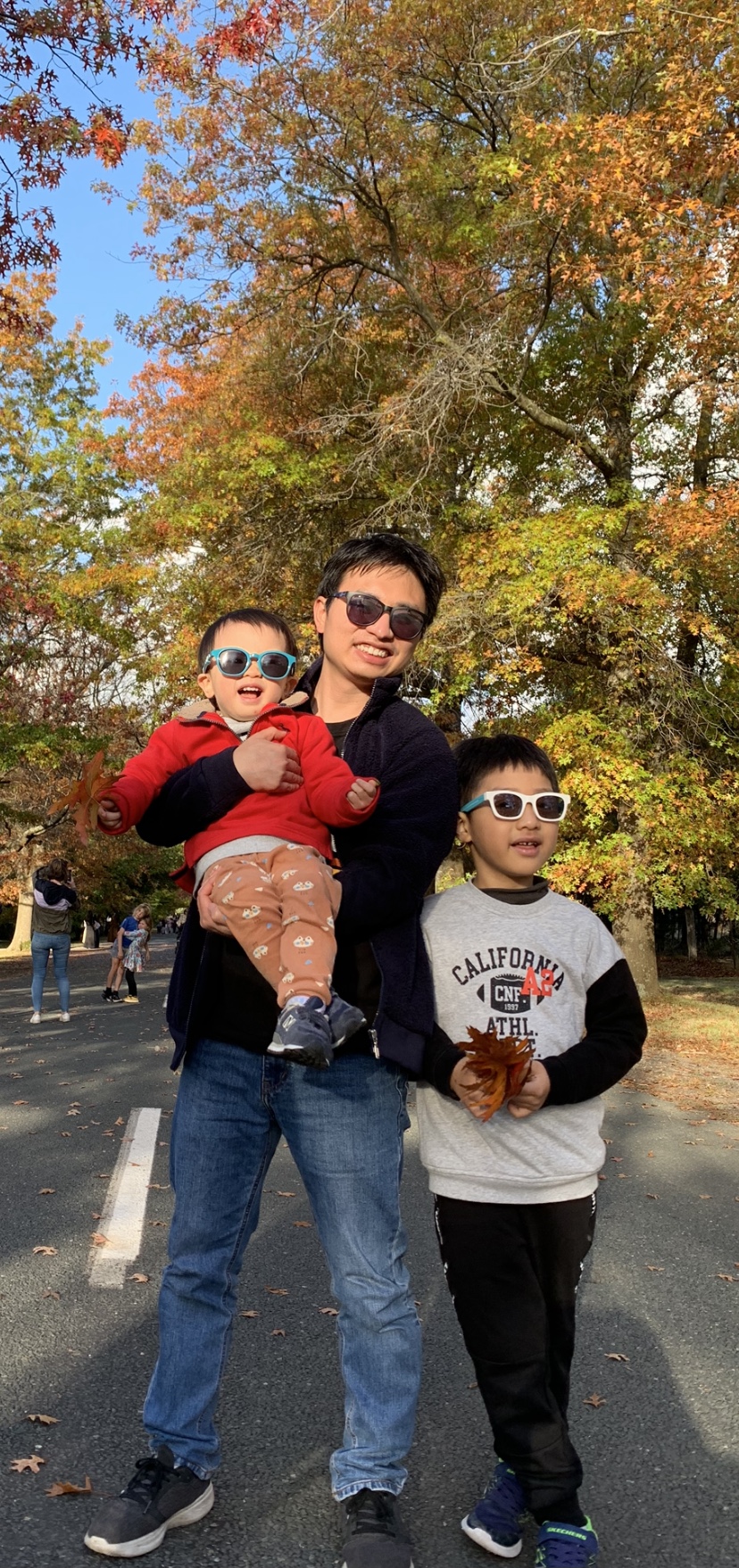
Me and two boys
I am Research Fellow at the Department of Data Science and AI, Monash University. My research interest lies in the intersection between Generative AI and Trustworthy Machine Learning. For example, my research focuses on how to ensure that models like ChatGPT do not respond to harmful queries asking to create a bomb, or that models like Stable Diffusion do not generate sexual images. I got my Ph.D. from Monash University in November 2023, under the supervision of Prof. Dinh Phung and Dr. Trung Le. My thesis can be found here.
Before that, I had one year working as Research Engineer at Credit AI Lab, Trusting Social, and two years working as Research Assistant at Singapore University of Technology and Design with Prof. Ngai Man Cheung and Dr. Trung Tran.
news
Jan 23, 2025 |
Hooray! I’m thrilled to finally share that our work has been accepted to ICLR 2025! This is more than just an acceptance—I’m truly proud that all reviewers recognized and appreciated the originality and creativity of our approach to concept unlearning, with a clear motivation and comprehensive experiments. The paper can be found here ![]() ![]() ![]() |
---|---|
Oct 4, 2024 |
Excited to share another paper that I am very proud of. This paper is an extension of our NeurIPS 2024 paper, where we dive deeper into the impact of erasing one concept to the others, but this time, we focus on the choice of target concepts. The paper can be found here. Our paper’s name was inspired by the movie “Fantastic Beasts and Where to Find Them”. Hopefully, the reviewers enjoy it as much as the movie ![]() |
Sep 26, 2024 | Proudly to share that our paper “Erasing Undesirable Concepts in Diffusion Models with Adversarial Preservation” has been accepted at NeurIPS 2024. We had a challenging rebuttal period, where we worked hard to address the feedback from some tough but silent reviewers. Fortunately, we had other reviewers who actively engaged with us, sought to understand our paper, and ultimately championed it. So, in this happy moment, I want to express my gratitude to the anonymous reviewers ❤️, as well as to my incredible collaborators from Monash and DST. We will soon update the paper with all the details and code. The paper can be found here with its slides. Hope you enjoy it. |
Jun 28, 2024 | I am thrilled and proud to see the Trustworthy Machine Learning project, on which I have been a key contributor since my PhD, being extended to a new 3-year project funded by the Department of Defense, Australia. The project will focus on various aspects of Trustworthy Generative Models, including alignment, safety, and robustness. This project is not only the first major grant on Generative AI in our DSAI department but also across the entire FIT at Monash University. 🎉 🎉 🎉 |
Nov 1, 2023 |
I officially become a Dr. today! My thesis “Enhancing Adversarial Robustness: Representation, Ensemble, And Distribution Approaches” is available here. Today is also my wedding anniversary ![]() |
latest posts
Jan 28, 2025 | DeepSeek-R1 |
---|---|
Jan 18, 2025 | LLM Series - Part 4 - How to Jailbreak LLMs |
Jan 17, 2025 | LLM Series - Part 3 - Build a Chatbot with Ollama |